Population Flux is a typology machine that measures the different population trends of people in rooms and how people tend to move in and out of rooms (individual/group) in order for viewers to see how busy rooms are at certain times. I built a machine that would save the relative times when people would enter a given room, and use a graphics API to visualize the data.
Check out the GitHub Repo here.
– Setup – [TLDR Shorter Setup Description in Discussion Section]
The entire operation only requires 3 terminal launches! (serial port reader, web socket connection, localhost spawning). A roadmap of the communication layout is provided below.
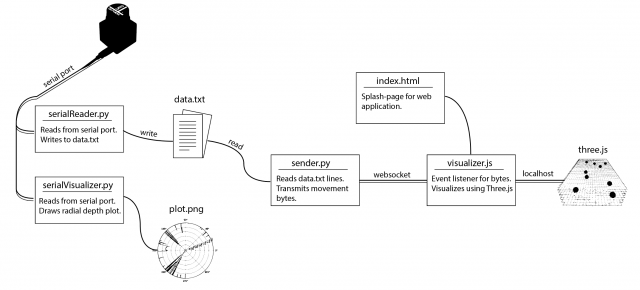
The involved setup was a Hokuyo URG Lidar connected to a laptop. The Lidar provided a stream of ~700 floats uniformly around 270 degrees at a rate of 10 times a second. In order to capture the data, I used the pyURG library to read directly from the serial port of the USB-hub where the Lidar was connected to. Unfortunately the interface is written for python2 and required substantial refactoring for python3 (the serial library that pyURG uses requires users to send bit-encoded messages with python3, which requires additional encoding/decoding schemes to get working with python3).
On startup, the program averages 10 captures in order to capture the room’s geometry. While the program reads from the Lidar, any data captured will have the initial room’s geometry subtracted from it so that all distances are negative (this is based on the assumption that the scene’s geometry does not change over time).
When a person walks at degree angle d in front of the Lidar, then region around d will return closer distances than before, and the resulting data after subtracting the scene’s geometry will be a wide negative peak at degree d. In order to get this exact location d, the data from each frame is sequentially parsed in an attempt to find the widest peak. Thus, at each frame, we can capture the location (in degrees) of any person walking in front of the Lidar.
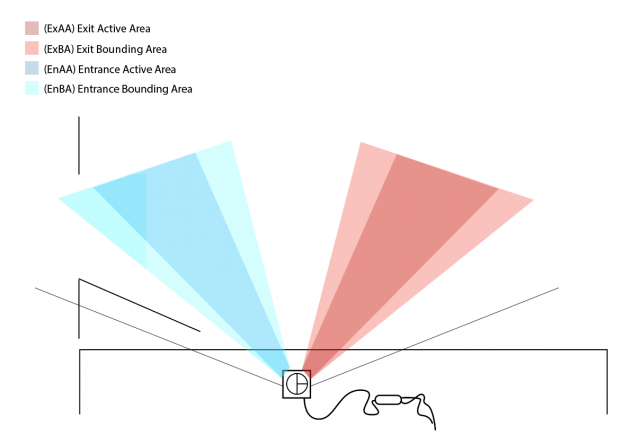
The next step is to determine the direction of movement, as it can be used to determine whether a person enters or exit a room. The Lidar’s view was discretized into 2 regions: the Entrance Active Area (EnAA) and Exit Active Area (ExAA), each containing a range of valid degrees that the area encased. When the user walked into EnAA, the program would push onto an entrance queue the timestamp of the detection, and when the user walked into ExAA, the program pushed the timestamp onto the exit queue. When both queues had at least one value on them, the first values were popped off and compared. If the enter timestamp was less than the exit timestamp, then the program would write the action as an “enter”. Otherwise, it would be written as an “exit”, along with the most recent timestamp of the two.
The biggest errors that would arise from this is getting multiple entrance timestamps added to the queue everytime a person stood in EnAA. To resolve this, a few steps were taken:
-
- Include booleans indicating if you are in EnAA or ExAA. The boolean would be turned on when first entering the area, and kept on to prevent multiple timestamps to be added to the queue.
- Add an Entrance Bounding Box (EnBA) Exit Bounding Box (ExBA) that were wider than EnAA and ExAA respectively. The booleans would only turn off when you exit these areas as to prevent multiple timestamps from being added to the queue if you’re on the edge of EnAA.
- Clear the queues once a person leaves the field of view of the Lidar. Originally if a person walks into EnAA, but turns around and leaves before reaching ExAA, then another person walks from the ExAA towards EnAA, then the program would misclassify it as an “enter” since it would compare the first EnAA timestamp of the person who turned around to the ExAA timestamp. Resetting the queue would prevent this issue.
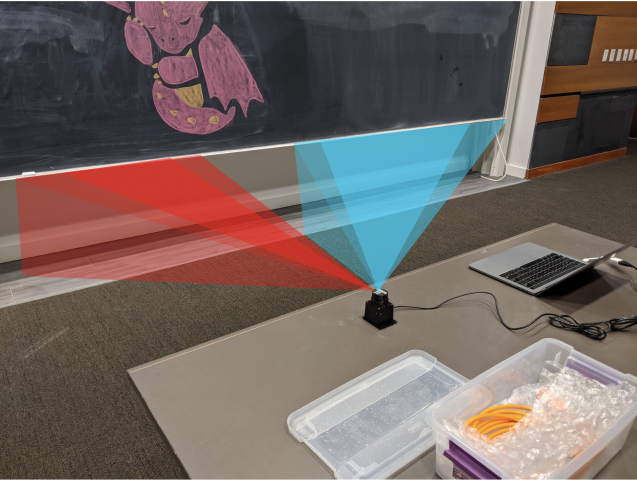
The resulting data was written to a text file in the form “[path,timestep]” where path was a boolean [0,1] indicating an enter or exit, and timestamp was the frame number in which the detection was made. Another python file would then read from the text file. It would then connect over web socket to the javascript visualization file and send over a series of bits denoting the number of enter and exit movements at any given timestep. The python script would do this by keeping track of the time of execution of the program, and anytime the timestamp of a particular datapoint was surpassed by the program’s time, then that point (0 for enter, 1 for exit) was added to the data stream and sent over web socket to the javascript file. This would allow users to replay pre-captured data in realtime, or speedup the playback by scaling up/down timesteps.
The javascript visualization was done in Three.js. Using a web socket listener, any incoming data would trigger the generation of new spheres that would be animated into the room. This way, viewers could see not only the number of people, but at what points people enter/exit the room, and if they move individually or all at once.
– Data –
The following data was captured over ~1-1.5 hour periods to measure the population traffic of rooms at different times.
Studio Space (Class-time) [30x speedup]
Studio Space (Night-time) [30x speedup]
– Discussion –
- What was your research question or hypothesis?
- What is the population density of a specific room at any given time? Do people tend to move in groups or individually? When are rooms actually free or available?
- What were your inspirations?
- This project was inspired by many of the building visualizations in spy movies and video games where you could find how dense certain rooms were at certain times.
- How did you develop your project? Describe your machine or workflow in detail, including diagrams if necessary. What was more complex than you thought? What was easier?
- The setup involved parsing incoming Lidar data to detect peaks, which would indicate the location of any passing people. The Lidar’s field of view was divided into an entrance and exit side, where if any peak passed through the entrance first, then it would classify the result as an “entrance”, otherwise an “exit”. The resulting data was stored in a text file and parsed by another python file before transferring over to a javascript visualization built on Three.js. The complex part was trying to get the data, as I was never able to get the OSC data outside of the receiver, so I ended up building my own layout for reading and transmitting the data. The easier part was getting the web socket set up considering it worked on the first try.
- In what ways is your presentation (and the process by which you made it) specific to your subject? Why did you choose those tools/processes for this subject?
- Determining if a person walks by isn’t very difficult, but determining the direction requires at least two datapoints. Luckily, Lidar offers more than 700 datapoints that can be divided into an entrance/exit region. This provides multiple points for each region, so if the subject moves quickly, the Lidar has a large field of view to detect the person. Three.js was used as the visualization scheme since it was easy to set up and convert to a web app.
- Evaluate your project. In what ways did you succeed, or fail? What opportunities remain?
- The project succeeded in being able to communicate the data from the Lidar all the way to the visualizer (and in real time!). This was overall tricky since there was a lot of processing done to the data, yet in the end there was a continuous stream of data with low latency being provided to the visualizer. One of the hardest part of the project was getting clear data from the Lidar. The device itself is very noisy, and I spent a lot of time trying to de-noise the output, but noise would still remain. One opportunity that still remained is advancing the visualization aspect. If I had more time, I would make a more complex room layout that would be more representative of the room geometry than just a green rectangle. This wouldn’t be difficult to do, just time consuming since it would require modeling.