‘Triple Chaser’ by Forensic Architecture
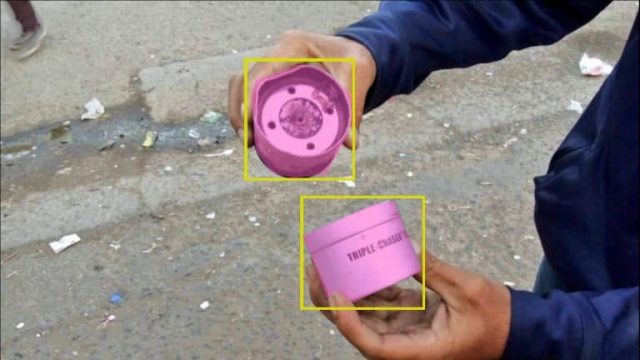
In 2018 the US fired tear gas canisters at civilians at the USA/Mexico Border and images identifying these munitions as ‘triple chaser’ tear gas grenades emerged. These are produced by the Safariland Group,which is owned by Warren B. Kanders who is the vice chair of the board of trustees of the Whitney Museum of American Art. Why mention all of this?
During the 2019 Whitney Biennial Exhibition, Forensic Architecture decided to create a camera that employed machine learning and computer vision techniques to scour the internet and determine in what conflicts (often states oppressing people) these tear gas canisters were being used. This information is not something easy to find and there were even not enough images of the canisters available to create an effective computer vision classifier, so they had to create models and make their own data so the classifier could work and then be used to identify real-world examples of where the were being used. The results and process of this investigation were then shown at the exhibition. They eventually withdrew the work from the exhibition in response to inaction on their findings.
What I love about this work is the use of inventive technology to create a solution for justice-oriented journalism/activism that would be impossible without the invention of the tools it crested. There would be no other way to sift through endless footage to put together a damning case of the widespread use of these tear gas devices and their relationship to a board member of the Whitney without the use of creating a process, or a performance, that can operate on its own. Also the idea of creating data needed to mine real world data in an effective way seems to be an interesting process for thinking about capture and how to deal with intentionally created blind spots. How to teach something to see as an act of art and activism?
Link to the video they created and presented is here.
Link to their methodology and explanation for creating the object identifier here.